Explainable Artificial Intelligence Will Help Develop Trust in AI Systems
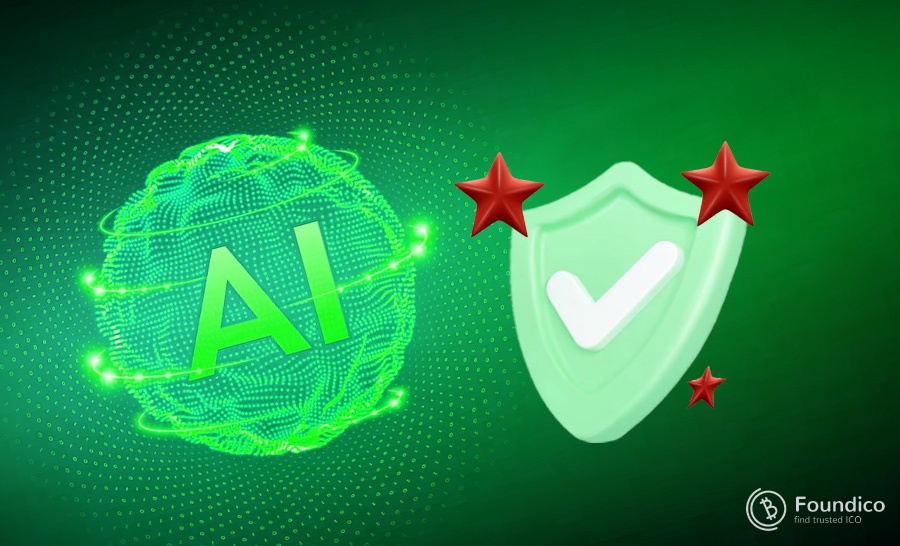
Our lives are rapidly changing as a result of artificial intelligence (AI), from healthcare and finance to transportation and entertainment. But as AI spreads, there are more and more worries about how many of these systems lack accountability and transparency. This has given rise to "explainable AI," which aims to make AI systems more transparent and comprehensible in order to increase stakeholder and user confidence.
AI systems are referred to as being "explainable" if they can give a human-understandable justification for their decisions and results. This is crucial in fields like healthcare, where AI is being used more and more to support decision-making regarding diagnosis and treatment. In these situations, doctors and patients need to be able to trust and rely on AI systems, so they must be aware of how the AI arrived at its conclusions.
The complexity of many AI systems is one of the main obstacles to creating AI that is understandable. For instance, deep learning algorithms may contain millions of parameters and layers, making it challenging to comprehend how the system came to a particular conclusion. In order to meet this challenge, researchers are creating novel methods for interpreting and visualizing AI systems, such as heatmaps and decision trees that let users see how the system arrived at its conclusions.
The need to strike a balance between performance, accuracy, and explainability presents another difficulty. The most accurate AI systems might not always be the easiest to understand. For instance, even a deep learning model that effectively predicts the course of a disease using genomic data may be challenging to understand. In order to balance explainability with accuracy and performance, researchers are working to create new techniques. One such technique is to create hybrid models that combine interpretable and non-interpretable AI components.
Additionally, explainable AI has social and ethical ramifications. Consider the use of AI for decision-making in the hiring, lending, and criminal justice processes, where biases and discrimination may be exacerbated by AI systems. By making AI decision-making transparent and accountable, explainable AI can aid in addressing these worries.
It is crucial to adopt a proactive and open approach to explainability in order to increase user confidence in AI systems. Involving users and stakeholders in the development process is one aspect of this, as is providing concise and understandable justifications for how AI makes decisions. A commitment to using AI ethically and responsibly is also necessary, as are actions to address bias and discrimination in AI systems.
In conclusion, explainable AI is a significant advancement in the field of artificial intelligence because it aims to make AI systems more transparent and comprehensible so that users and stakeholders can have faith in them. The potential benefits of creating explainable AI outweigh the challenges, which include the complexity of many AI systems and the need to strike a balance between explainability, accuracy, and performance. We can develop AI systems that are more dependable, responsible, and ethical, and that can assist in addressing some of the most urgent issues facing society today, by adopting a proactive and open approach to explainability.
An Analysis by Pooyan Ghamari, Swiss Economist with Expertise in the Digital World